September 2021: Advanced Intelligent Systems
Bayesian Optimization (BO) has emerged as the algorithm of choice for driving autonomous high throughput experiments. However, some recent theoretical and experimental studies cast doubt on the efficiency of the default Gaussian Process (GP) surrogate model, especially for optimization of complex datasets. Here, we performed a systematic optimization study using simulated experiments, and compared various surrogate models for BO including GP and also Neural Network Ensemble. We found that with proper choice of the GP kernel and parameters, the GP surrogate model is still the most efficient, allowing the BO to extrapolate fastest towards the optimum after 100 experimental iterations.
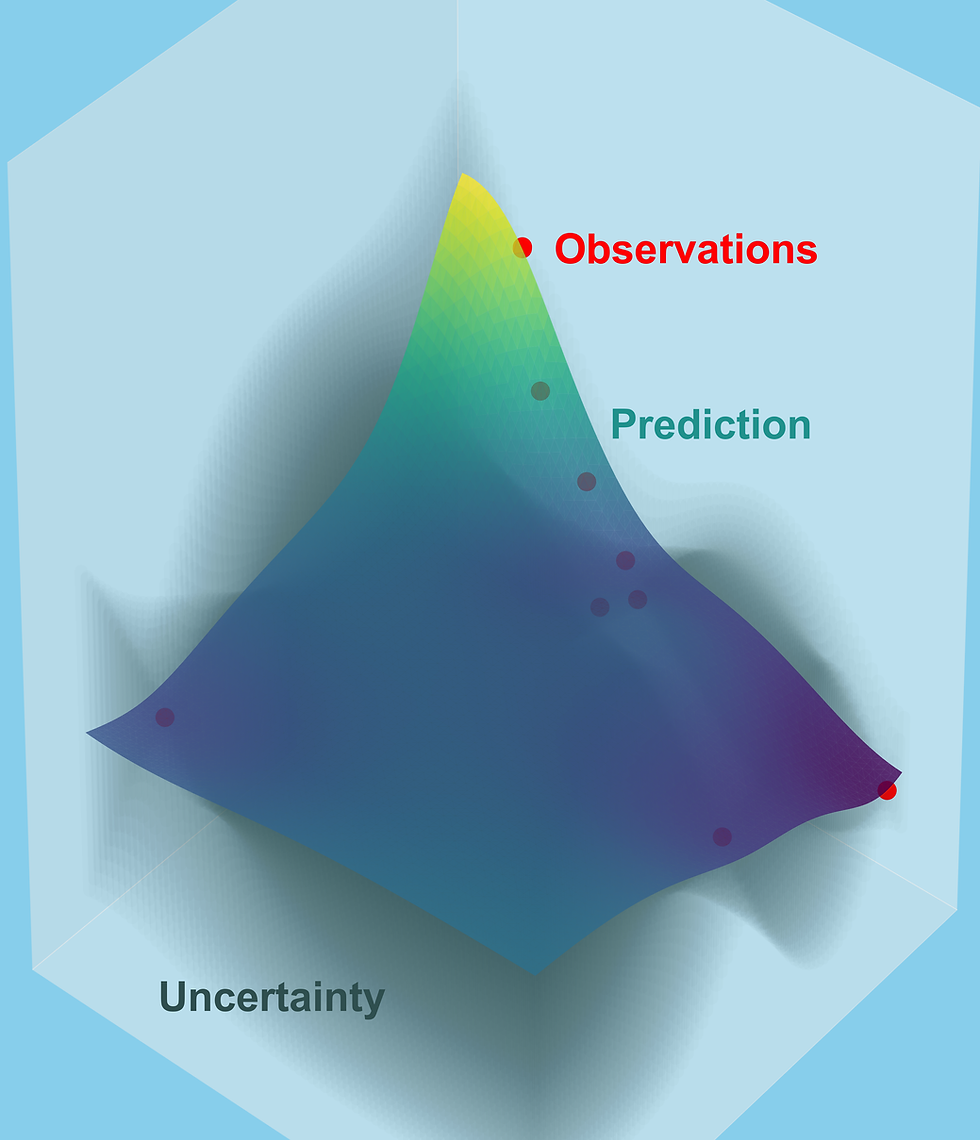
Comments