June 2021: Advanced Functional Materials
Combining high-throughput experiments with machine learning accelerates materials and process optimization toward user-specified target properties. In this study, we developed a high-throughput, machine learning-driven mixing and drop-casting system to fabricate the films, as well as tools and procedures for rapid proxy measurements of electrical and optical properties of these films. In the end we were able to make 160 composites of poly-3-hexylthiophene and various types of nanotubes per day, which is more than 10x improvement compared to baseline. Graph-based model selection strategies with classical regression, in combination with Bayesian Optimization, was used to suggest next experiments to try and predict their performance. In 12 cycles of experiments, the ML predictions and measured results converged, yielding the optimal composition of a target material, with state-of-the-art conductivity of 1000 S/cm. These results were later verified with manual high-fidelity experiments. These strategies present a robust machine-learning driven high-throughput experimental scheme that can be effectively applied to understand, optimize, and design new materials and composites.
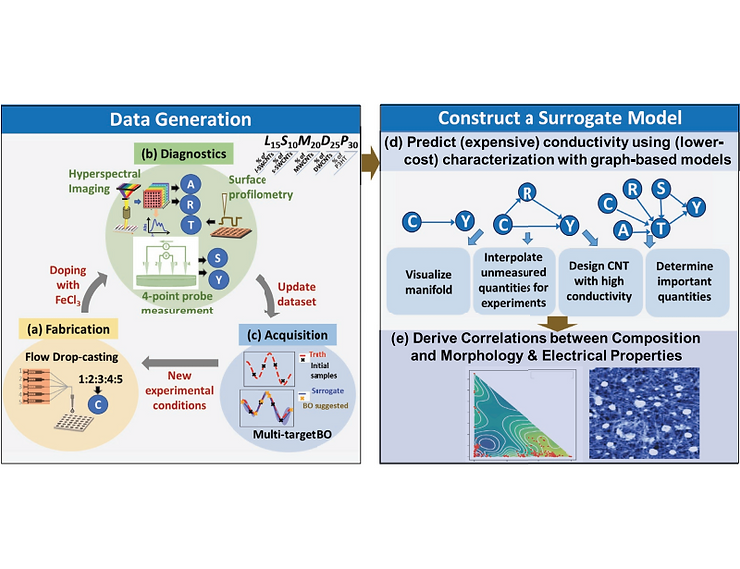
Comentários