Dr. Apurva Mehta (31 March 2021)
- Cynthia Chew
- Mar 30, 2021
- 2 min read
Topic: Data-driven Approaches to Accelerated Discovery of Complex and Metastable Materials
Speaker: Dr. Apurva Mehta from SLAC National Accelerator Laboratory
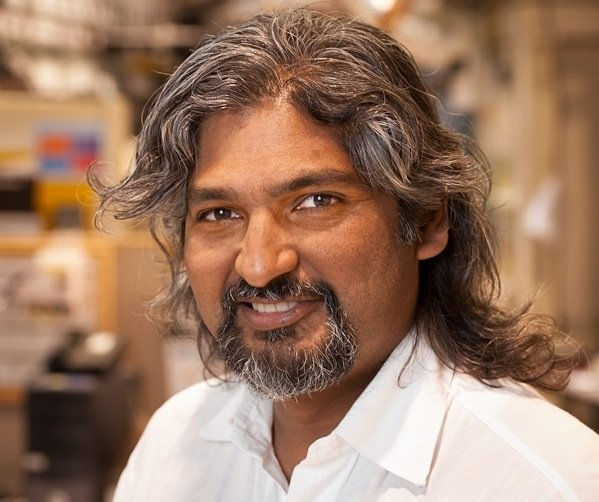
With great joy, we host Dr. Apurva Mehta from SLAC National Accelerator Laboratory this week to outline a combination of machine-learning and AI-directed (high throughput) experiments to accelerate discoveries of structural and functional multi-principal-element alloys.
Abstract
The complex material systems are beginning to play a growing role in addressing some of our current technological challenges. They include multi-principal element alloys and oxides (often also called high entropy alloys/oxides), metastable materials like metallic glasses and carbon nanotubes, solid-state energy storage devices, heterogeneous catalysts, and increasingly complex devices for power electronics and quantum computing. However, there are easily tens of million and possibly even a billon compositions out of which a very small fraction are potential candidates. Many of the desired materials are metastable and therefore processing paths play a crucial role in synthesizing them. The composition-processing combinatorial search space is too vast for a dependence on serendipitous discoveries, to provide urgently need novel materials and devices; and a brute-force survey of the combinatorial space is too slow and expensive.
We need guidance to navigate the vast composition-processing space. Our physiochemical understanding of complex material systems is still too nascent to provide it. Recent advances in machine-learning (ML) and other AI-tools, however, suggests that a data-driven approach that builds up on insights from physiochemical theories, and bridges the gaps in the insights by experimental observations can provide the needed navigation. Application of ML to large experimental observations can highlight hidden and complex trends that theories may miss. These predictions also come with uncertainty quantification. The combination of predictions and uncertainties allow us to device different experimental strategies, ranging from exploitive to exploratory to help direct us to the next high impact experiment to perform, leading to accelerated discoveries and new physiochemical insights. Here, I will demonstrate these approaches using examples from amorphous and crystalline Multi-Principal-Element Alloys with desired structural and thermoelectric properties.
Biography
As the lead scientist at SLAC, he has been leading the effort of high-throughput structural characterization of new inorganic materials at the beamline, with machine learning, computations and optimization in the loop.
Very much looking forward to the seminar – hope to see you all on Wednesday: (SGT) 9am, Wed, 31 Apr! https://mit.zoom.us/j/96231985116
Warmest regards, @Siyu Tian (Isaac) @kedar @Tonio
Comentários