Topic: Amortized Tree Generation for Bottom-up Synthesis Planning and Synthesizable Molecular Design.
Speaker: Mr. Wenhao Gao from Department of Chemical Engineering at Massachusetts Institute of Technology (MIT)
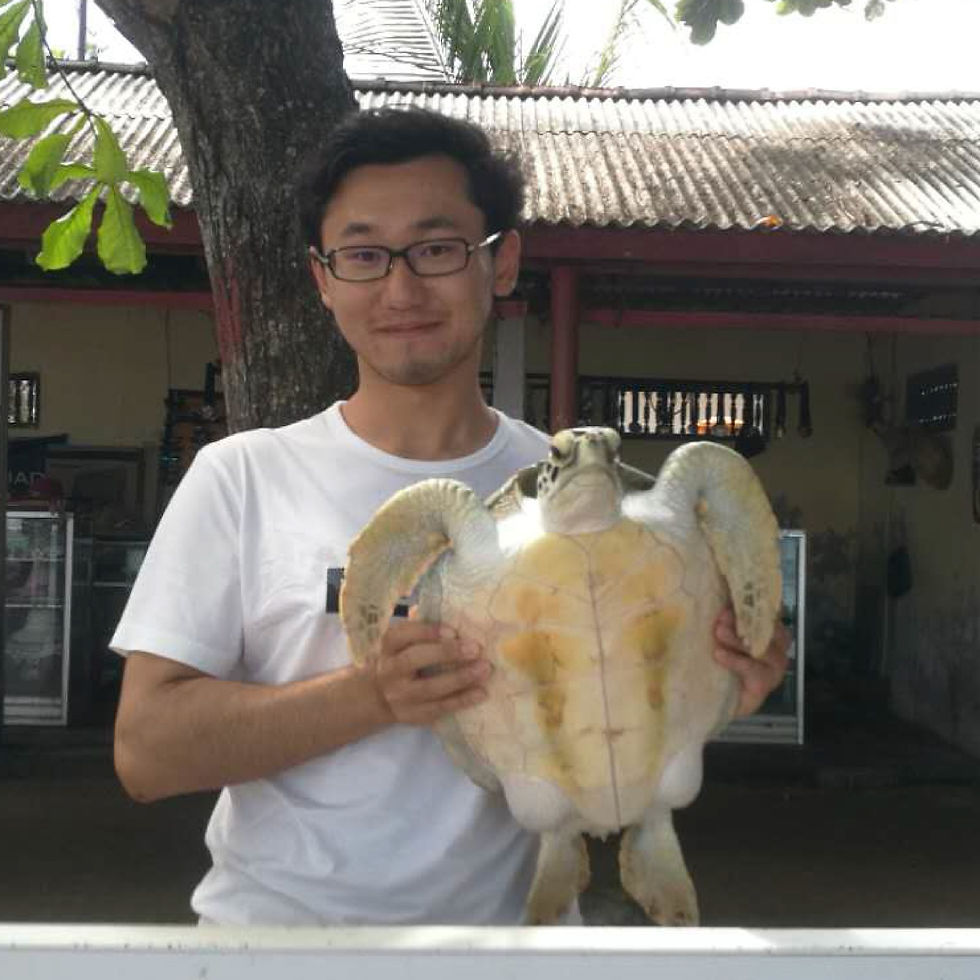
We’re super delighted to announce our next speaker, Mr. Wenhao Gao, from Department of Chemical Engineering at Massachusetts Institute of Technology (MIT), to bring us:
“Amortized Tree Generation for Bottom-up Synthesis Planning and Synthesizable Molecular Design”
Abstract
Molecular design and synthesis planning are two critical steps in the process of molecular discovery that we propose to formulate as a single shared task of conditional synthetic pathway generation. We report an amortized approach to generate synthetic pathways as a Markov decision process conditioned on a target molecular embedding. This approach allows us to conduct synthesis planning in a bottom-up manner and design synthesizable molecules by decoding from optimized conditional codes, demonstrating the potential to solve both problems of design and synthesis simultaneously. The approach leverages neural networks to probabilistically model the synthetic trees, one reaction step at a time, according to reactivity rules encoded in a discrete action space of reaction templates. We train these networks on hundreds of thousands of artificial pathways generated from a pool of purchasable compounds and a list of expert-curated templates. We validate our method with (a) the recovery of molecules using conditional generation, (b) the identification of synthesizable structural analogs, and (c) the optimization of molecular structures given oracle functions relevant to drug discovery.
Biography
Wenhao Gao is a Ph.D. candidate in Chemical Engineering at MIT. Under advisory from Prof. Connor Coley, his research focuses on using artificial intelligence and robotic automation to accelerate chemical discovery processes. Before joining the Coley research group, he received his B.S. in Chemistry from Peking University and M.S. in Chemical and Biomolecular Engineering from Johns Hopkins University.
Very much looking forward to the seminar – hope to see you all on Wednesday: (SGT) 9am, Wed, 22 December! https://mit.zoom.us/j/96231985116