January 2022: Digital Discovery
Functional composite thin films have a wide variety of applications in flexible and/or electronic devices, telecommunications and multifunctional emerging coatings. Rapid screening of their properties is a challenging task, especially with multiple components defining the targeted properties. In this work we present a manifold for accelerated automated screening of viscous graphene suspensions for optimal electrical conductivity. Using Opentrons OT2 robotic auto-pipettor, we tested 3 most industrially significant surfactants - PVP, SDS and T80 - by fabricating 288 samples of graphene suspensions in aqueous hydroxypropylmethylcellulose. Enabled by our custom motorized 4-point probe measurement setup and computer vision algorithms, we then measured electrical conductivity of every sample and identified that the highest performance is achieved for PVP-based samples, peaking at 10.4 mS/cm. The automation of the experimental procedure allowed us to perform the majority of the experiments using robots, while involvement of human researchers was kept to minimum. Overall the experiment was completed in less than 18 hours, only 3 of which involved humans.
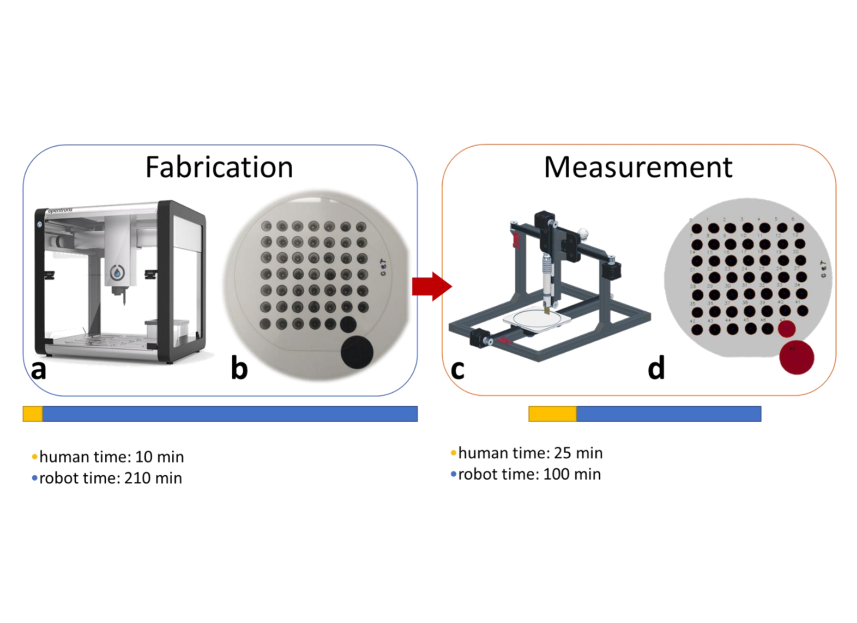