August 2018 - Joule : Cell Press
In collaboration with Prof. Tonio Buonassisi (MIT), we lay out the pathway for increasing the speed of discovery.
Successful materials innovations can transform society. However, materials research often involves long timelines and low success probabilities, dissuading investors who have expectations of shorter times from bench to business. A combination of emergent technologies could accelerate the pace of novel materials development by ten times or more, aligning the timelines of stakeholders (investors and researchers), markets, and the environment, while increasing return on investment. First, tool automation enables rapid experimental testing of candidate materials. Second, high-performance computing concentrates experimental bandwidth on promising compounds by predicting and inferring bulk, interface, and defect-related properties. Third, machine learning connects the former two, where experimental outputs automatically refine theory and help define next experiments. We describe state-of-the-art attempts to realize this vision and identify resource gaps. We posit that over the coming decade, this combination of tools will transformthe way we perform materials research, with considerable first-mover advantages at stake.
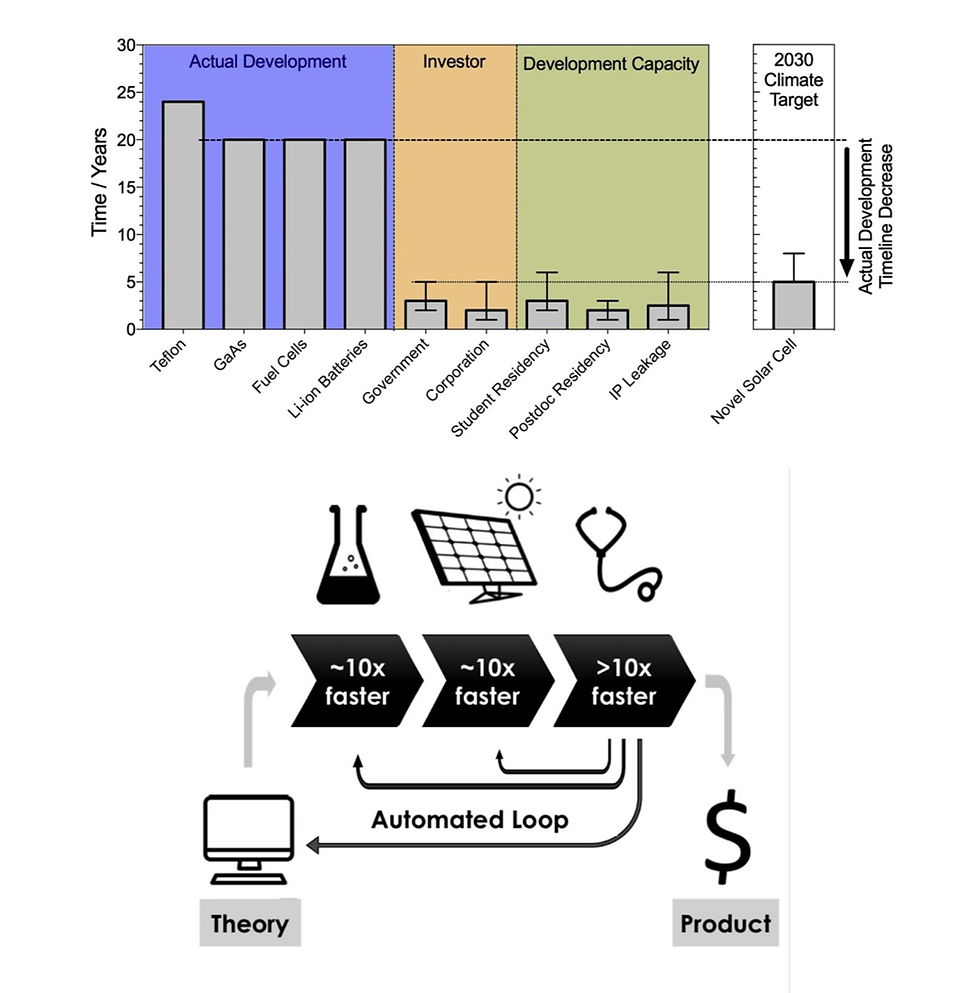