Topic: Using statistical learning and the Physics of image formation to evolve exploratory microscopy
Speaker: Dr. Duane Loh from National University of Singapore
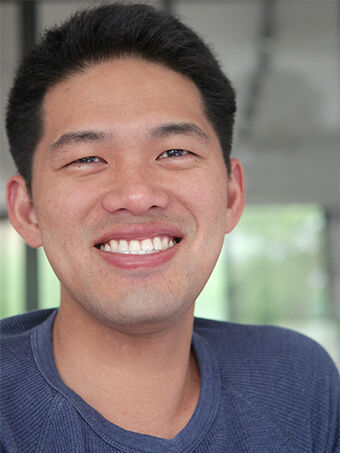
We’re super delighted to host Dr. Duane Loh from National University of Singapore to share with us:
“Using statistical learning and the Physics of image formation to evolve exploratory microscopy”
Duane Loh has been a thought leader in the AI in science field in Singapore! his talk is long-awaited! He’s also an awesome speaker!
Abstract
The evolution of human vision is a fascinating interaction between our eyes and brains. At the very beginning, so the story goes, variations of primitive eyes receive input that is interpreted by simple yet plastic neural networks that in turn trigger responses. When these responses confer the organism with evolutionary fitness, the causal “wetware” (biological equivalent of hardware) and “software” are selectively propagated to the progeny. Iterating such selective propagations over many generations, purportedly, created the powerful visual perception that you are using to read this abstract.
A different type of interaction between hardware and software also occurs in the iterative evolution of microscopy. Humankind has a storied history of inventing many novel forms of microscopy using visible light, x-rays, and electrons. Each invention, however wild or incremental, produced images that fed the development of Physical theories of image formation, which in turn empowered the design of the next generations of microscopy.
Generations of such iterative design later, it is often impossible for humans to interpret the raw micrographs without using the knowledge from the theory of image formation. This is especially true when the imaging setup only permits us a very noisy and incomplete view of the sample. In these scenarios, the combination of statistical machine learning with the theory of image formation is critical.
In this talk, I will describe how my group has been combining the Physics of image formation with statistical learning to create statistical lenses for high-resolution microscopy. These combinations, we hope, will create vistas to explore novel, complex phenomena in the fragile, fleeting, and chaotic nanometer world.
Biography
Duane Loh is a faculty member in the departments of Physics and Biological Sciences at NUS. His is interested in combining machine learning with scientific and instrument priors to create computational lenses that help make sense of the chaotic and nearly invisible dynamics that occur at the nanometer-scale. Duane did his Bachelor of Science at Harvey Mudd College, specializing in Theoretical and Mathematical Physics. He would go on to earn his PhD in Physics from Cornell University for his feasibility studies of single-particle diffractive imaging with extremely noisy, and incomplete data. Thereafter, he moved to SLAC National Accelerator Laboratory at Stanford University, the world’s first hard X-ray Free-electron laser, to help realize these single-particle diffractive imaging experiments. At SLAC, Duane pioneered unsupervised machine learning to discover transient intermediate states in highly heterogeneous and dynamic phenomena. In 2013, he joined NUS as a Lee Kuan Yew post-doctorate fellow, where he extended these methods to electron microscopy to study dynamic order-disorder phenomena at the nanometer-scale. He started his research group NUS in 2016, and continues to develop core ideas and technologies towards far-reaching imaging modalities that are too challenging for hardware-based microscopy alone.
Very much looking forward to the seminar – hope to see you all on Wednesday: (SGT) 9am, Wed, 28 Apr! https://mit.zoom.us/j/96231985116
Warmest regards, @Siyu Tian (Isaac) @kedar @Tonio