Topic: Defects in Functional Materials: Doping, Discovery, and Dreams.
Speaker: Dr. Prashun Gorai from the Colorado School of Mines
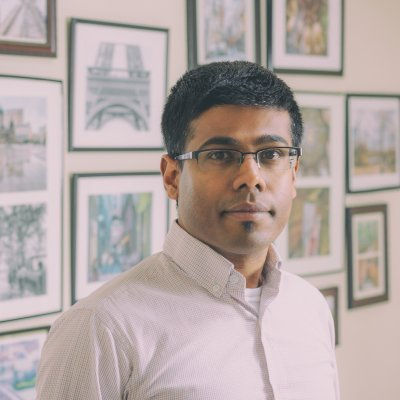
This is a long awaited talk! We’re chuffed to announce our next speaker, who’s dreaming about defects, doping, DFT and machine learning Dr. Prashun Gorai from the Colorado School of Mines, to bring us:
“Defects in Functional Materials: Doping, Discovery, and Dreams”
Abstract
Defects play a critical role in influencing the functional properties of materials in applications such as thermoelectrics, optoelectronics, solid-state energy storage etc. In this talk, I will give a brief overview of first-principles modelling of point defect energetics and software packages for automation of such calculations. I will provide examples from our work on thermoelectrics, photovoltaics, and solid-state batteries, where we have utilized first-principles defect calculations to guide experimental doping efforts, enable discovery of new materials and develop new composition-structure-property relationships. I will also introduce our recent work on a doping by design approach to materials discovery, and extension of defect modelling to disordered phases. In the end, I will share my vision of how materials informatics can accelerate dopability predictions and therefore, materials discovery. In this context, I will discuss the need for a standardized data format for documenting defect calculations.
Biography
Dr. Prashun Gorai is a research assistant professor in the Department of Metallurgical and Materials Engineering at the Colorado School of Mines (CSM). He is also affiliated with the Materials Science Center at the National Renewable Energy Laboratory (NREL). He obtained his BTech degree from the Indian Institute of Technology Madras and his PhD from the University of Illinois at Urbana-Champaign. Subsequently, he was a postdoctoral fellow at CSM and NREL. Dr. Gorai’s research team uses computational tools, including first-principles methods and materials informatics, to discover and design functional materials for various energy-related applications.
Very much looking forward to the seminar – hope to see you all on Wednesday: (SGT) 9am, Wed, 4 August! https://mit.zoom.us/j/96231985116